We are committed to protecting your personal data and providing you with access to your personal data in accordance with the personal data protection laws in force in the European Union. We have updated and will periodically update our privacy policy to comply with this personal data protection law. Please refer to the latest version of our Privacy Statement.
This website uses cookies to provide a better browsing experience. To learn more about how cookies are used on this site please click here.
17.01
2025
From the Laboratory to the Production Line: Spingence Collaborates with National Central University to Accelerate Industrial AI Adoption
From the Laboratory to the Production Line: Spingence Collaborates with National Central University to Accelerate Industrial AI Adoption
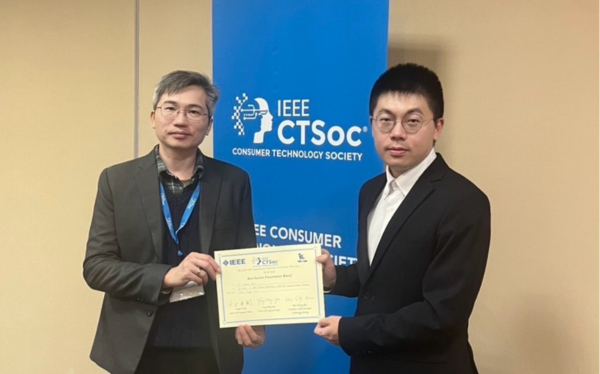
(Photo: Hsu Jen-Chueh, received the Best Session Presentation Award at the IEEE International Conference on Consumer Electronics (ICCE 2025). Source: Hsu Jen-Chueh)
Spingence has partnered with Professor Po-Chyi Su and graduate student Jen-Chueh Hsu from the Department of Computer Science and Information Engineering at National Central University to develop an innovative "Defect-Aware Segmentation Model (DA-SAM)." This groundbreaking technology overcomes multiple technical barriers in traditional industrial defect detection. The collaboration not only provides academia with a practical and versatile solution but also opens new directions for quality inspection in manufacturing. The research was published at a prestigious IEEE conference and showcased at ICCE 2025, held from January 11 to 14 in Las Vegas, USA.
Breaking Barriers in Industrial Defect Detection: The Innovation and Potential of DA-SAM
ndustrial defect detection is one of the core challenges in manufacturing quality control. While traditional methods like Automated Optical Inspection (AOI) can achieve a certain level of efficiency in stable environments, their lack of adaptability struggles to meet increasingly complex and variable production conditions.
This leads to significant time and cost burdens for manufacturers in calibration and maintenance. Although AI offers promising advantages, modern manufacturing's high yield rates make collecting negative samples challenging, further extending the project evaluation and implementation timeline.
To address these issues, Spingence and National Central University developed the DA-SAM model. By combining the Segment Anything Model (SAM) with Low-Rank Adaptation (LoRA) technology, this high-efficiency deep learning tool significantly enhances detection performance while reducing labeling time. Experimental results show that DA-SAM achieves an accuracy of 76.1% with just five samples, demonstrating strong few-shot learning capabilities and providing an effective solution to the challenge of insufficient negative samples.
Additionally, the research team released a new industrial defect dataset called SPTD. Compared to the MVTecAD dataset, SPTD offers more precise, small-scale defect samples tailored to industrial contexts, making it valuable for both academic research and practical applications in manufacturing.
This leads to significant time and cost burdens for manufacturers in calibration and maintenance. Although AI offers promising advantages, modern manufacturing's high yield rates make collecting negative samples challenging, further extending the project evaluation and implementation timeline.
To address these issues, Spingence and National Central University developed the DA-SAM model. By combining the Segment Anything Model (SAM) with Low-Rank Adaptation (LoRA) technology, this high-efficiency deep learning tool significantly enhances detection performance while reducing labeling time. Experimental results show that DA-SAM achieves an accuracy of 76.1% with just five samples, demonstrating strong few-shot learning capabilities and providing an effective solution to the challenge of insufficient negative samples.
Additionally, the research team released a new industrial defect dataset called SPTD. Compared to the MVTecAD dataset, SPTD offers more precise, small-scale defect samples tailored to industrial contexts, making it valuable for both academic research and practical applications in manufacturing.
From Academia to Industry: Far-Reaching Impact and Future Outlook
The international academic recognition of this research stems not only from its technical innovation and practicality but also from Spingence's extensive experience in manufacturing applications. As a leading industrial AI company, Spingence deeply understands the pain points of visual inspection in industrial settings. The company drives innovation and breakthroughs with a focus on meeting the most stringent inspection requirements.
Spingence emphasized that this collaboration accelerates its technological advancements in industrial AI and lays a solid foundation for future product development and customer service. The company remains committed to reducing the cost and barriers for manufacturers to adopt AI, focusing on providing efficient, reliable, and cost-effective solutions to help the industry achieve intelligent upgrades.
Looking ahead, Spingence will deepen its collaborations with academic institutions, addressing core challenges in visual defect detection and driving advancements in global industrial inspection technologies.
About Spingence
Spingence emphasized that this collaboration accelerates its technological advancements in industrial AI and lays a solid foundation for future product development and customer service. The company remains committed to reducing the cost and barriers for manufacturers to adopt AI, focusing on providing efficient, reliable, and cost-effective solutions to help the industry achieve intelligent upgrades.
Looking ahead, Spingence will deepen its collaborations with academic institutions, addressing core challenges in visual defect detection and driving advancements in global industrial inspection technologies.
About Spingence
Spingence is a software company specializing in AI defect detection and automation for the manufacturing industry, offering one-stop solutions. The AINavi platform's core product supports the entire workflow from labeling to training and deployment. The platform has been successfully implemented in semiconductors, passive components, connectors, and optical lens modules, earning trust and recognition from the market.